In the world of cardiac electrophysiology (EP), we love getting into deep discussions about tiny waves, complexes, and patterns. Despite our experienced “eyeball calipers” and collective years of staring at endless screens of squiggly lines, we oftentimes arrive at different interpretations. And forget about adding in patient presentation – disease state, arrhythmia mechanism, frequency of events, pharmacological considerations, past medical history, etc. How can we possibly expect to have consistent and reproducible results in our treatment plan?
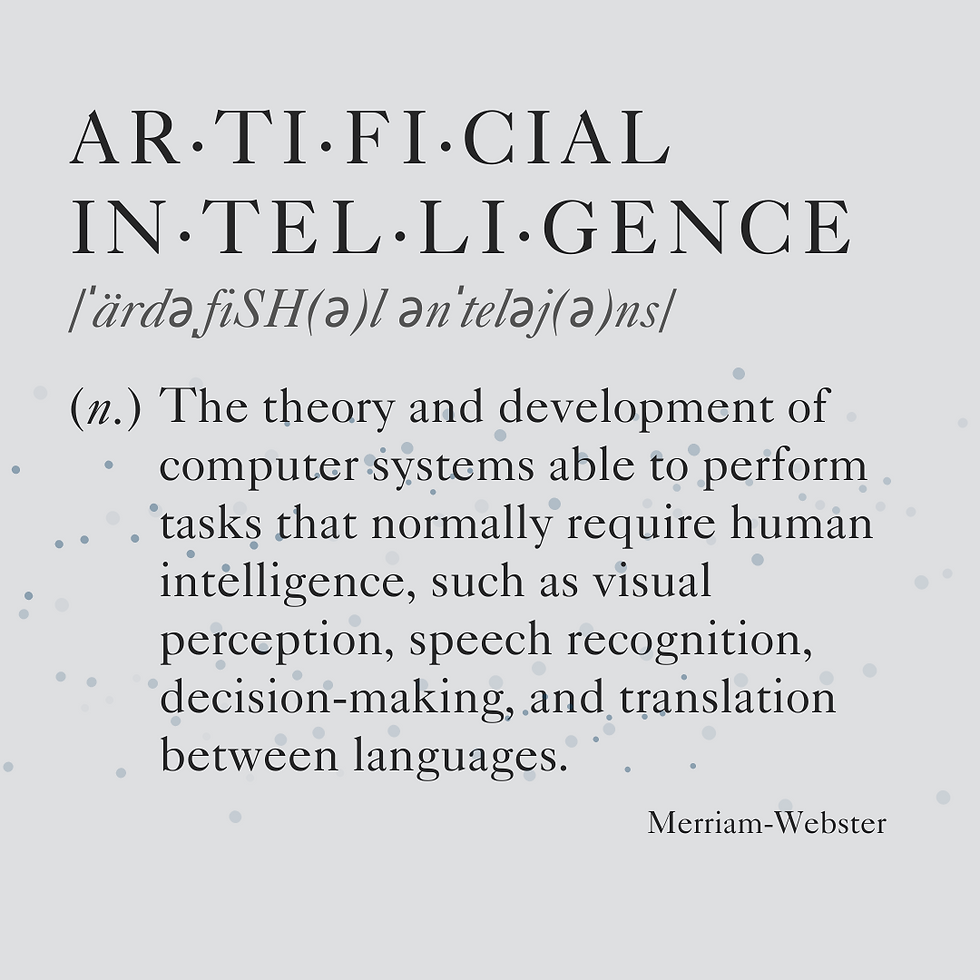
Thinking Differently
Artificial Intelligence (AI) is no longer a futuristic concept; it’s a reality that's reshaping healthcare. In EP, AI brings powerful tools to our fingertips, enhancing our ability to diagnose, treat, and manage cardiac arrhythmias. So, how does AI think differently?
Let’s review the two primary categories of AI:
Machine Learning (ML): Think of ML as the seasoned detective, sifting through historical patient data to predict arrhythmia risks, classify ECG/EGM signals, and forecast outcomes. ML models learn from past cases to provide insights that can be pivotal in-patient care.
Deep Learning (DL): This is the brainiac of AI, using neural networks to delve into complex data structures. DL shines in interpreting imaging data from MRIs and CT scans, offering detailed cardiac maps that reveal subtle anomalies, aiding in precise diagnosis.
What Data…and How Much?
In the field of EP, the quality and quantity of data are two crucial factors for developing effective and ethical AI models – Data Quality and Quantity. It is important to strike a balance between these two aspects to create accurate and reliable AI models that can provide robust solutions to healthcare problems.
DATA QUALITY emphasizes the accuracy, completeness, and reliability of the information. It ensures that the data used for training and validation are error-free and representative of real-world scenarios.
Importance in AI:
Model Performance: High data quality contributes to the performance of AI models by ensuring that the patterns learned during training reflect the true relationships in the data.
DATA QUANTITY refers to the volume of data available for model training. It's concerned with having a sufficiently large dataset to capture diverse patterns and variations.
Importance in AI:
Generalization: A larger dataset helps the model generalize well to diverse cases, reducing the risk of overfitting to specific patterns present in a smaller dataset.

Importance of Data Quality and Quantity in EP
Accurate Model Training: The success of AI models in EP heavily relies on the quality of the data used for training. High-quality data ensures that the model learns from accurate, representative examples, improving its ability to make reliable predictions and diagnoses.
Generalization to Diverse Cases: Quality data contributes to the model's ability to generalize well across diverse patient populations and cases. This is crucial in EP, where individual variations in patient data are significant. Robust models can handle variations and nuances present in real-world scenarios.
Patient Safety and Outcome Improvement: Accurate and high-quality data lead to more reliable AI-driven predictions and recommendations. In cardiac electrophysiology, where decisions directly impact patient safety and outcomes, the reliability of AI models becomes paramount.
What’s So Complex About a Complex?
In complex domains like electrophysiology, a larger dataset can help the model handle the intricacies and variations present in different patients and conditions.
Integrating AI in Electrophysiology is Complex...
1. Balancing Act: Achieving an optimal balance between data quality and quantity is crucial. A large dataset with poor quality may lead to unreliable models, while a high-quality dataset with insufficient quantity may limit the model's ability to generalize.
2. Ethical Considerations: As datasets become larger, ethical considerations related to privacy, consent, and security become even more critical. Achieving a balance between data quantity and ethical considerations is essential in AI applications.
3. An Iterative Process: Building datasets is often an iterative process. Continuous refinement, validation, and augmentation are necessary to improve both the quality and quantity of the dataset.
Challenges:
1. Data Heterogeneity: Arrhythmia data comes from various sources, including ECGs, imaging, patient records, and even wearables. Integrating heterogeneous data types and ensuring consistency pose challenges in maintaining data quality.
2. Data Privacy and Security: Healthcare data, especially in cardiology, is sensitive and subject to privacy regulations. Balancing the need for access to data for model training with the imperative to protect patient privacy is a delicate challenge
3. Data Annotation and Labeling: High-quality data often requires accurate annotation and labeling. In EP, where precise identification of arrhythmias and other cardiac conditions is crucial, obtaining accurately labeled data can be labor-intensive and prone to human error.
4. Limited Availability of Annotated Data: Obtaining large volumes of well-annotated data can be challenging due to the specialized nature of the field. The limited availability of labeled data may hinder the development of highly accurate models.
5. Bias in Training Data: If the training data is not representative of the diverse patient population, the AI model may learn biased patterns. This can result in disparities in diagnosis and treatment recommendations, especially for underrepresented demographic groups.
Gametime Decisions
Artificial Intelligence (AI) technology is not designed to replace healthcare professionals. Instead, it is meant to enhance their abilities, provide decision-making support, and ultimately improve patient outcomes. Imagine being able to detect cardiac conditions before they escalate. AI makes this possible by enabling early detection, precise risk assessment, and continuous monitoring of chronic conditions. As an example, wearable devices powered by AI can track heart rhythms in real-time, alerting both patients and clinicians to potential issues and ensuring timely intervention.
During procedures like catheter ablations, AI can offer real-time decision support, especially in complex procedures such as atrial fibrillation and ischemic ventricular arrhythmias. By analyzing live data, AI can suggest catheter navigation, predict potential complications, and recommend procedural adjustments, which enhance safety and efficacy. Outside of the procedure, AI-driven scheduling and resource allocation streamline workflows, reducing wait times and improving lab efficiency.

“Think left and think right and think low and think high. Oh, the things you can think up if only you try!” – Dr. Seuss
Oh, the Places We’ll Go…the Future of AI in EP
Cue the Dr. Seuss fans! “We have brains in our heads and patients to treat. The AI mountain is waiting, now let’s help their heartbeat. There is so much ahead for us to carefully explore. If we begin to imagine the capabilities of AI support in daily practice, there are endless possibilities.”
Below are a few that are on the horizon.
Improved Diagnosis and Risk Stratification: AI algorithms can analyze vast amounts of patient data, including ECGs, imaging studies, genetic information, and clinical records, to detect patterns and anomalies that may be missed by human interpretation alone. This can lead to more accurate and timely diagnosis of cardiac arrhythmias, as well as risk stratification for better patient management.
Enhanced Treatment Planning: AI can assist in personalized treatment planning by analyzing patient-specific data and recommending optimal treatment strategies. This includes selecting the most appropriate antiarrhythmic medications, suggesting the ideal ablation targets, or even predicting the likelihood of treatment success. AI-based treatment planning can improve outcomes and reduce procedural complications.
Real-time Decision Support: During procedures such as catheter ablation or device implantation, AI algorithms can provide real-time decision support to guide physicians. By integrating with imaging and mapping systems, AI can help identify critical structures, optimize electrode placement, and enhance procedural precision. This can result in improved procedural outcomes and reduced procedure times.
Predictive Analytics and Risk Assessment: AI can analyze large datasets to identify risk factors associated with adverse cardiac events, such as sudden cardiac death or post-procedural complications. By leveraging machine learning algorithms, AI can provide predictive analytics to help clinicians identify high-risk patients and implement preventive strategies, ultimately reducing morbidity and mortality.
Optimization of Workflow and Resource Allocation: AI algorithms can optimize workflow in cardiac electrophysiology departments by automating repetitive tasks, streamlining data analysis, and prioritizing patient appointments based on clinical urgency. This allows healthcare professionals to allocate their time and resources more efficiently, improving patient throughput and reducing waiting times.
Advanced Research and Innovation: AI has the potential to accelerate research and innovation in cardiac electrophysiology. By analyzing large-scale datasets, AI algorithms can uncover new insights, identify novel biomarkers, and contribute to the development of innovative diagnostic and therapeutic approaches. AI can also facilitate data sharing and collaboration among researchers, fostering advancements in the field.
Conclusion
Artificial Intelligence (AI) technology is not designed to replace healthcare professionals. Instead, it is meant to enhance their abilities, provide decision-making support, and ultimately improve patient outcomes.
Healthcare professionals will continue to play a crucial role in interpreting the results generated by AI, exercising their clinical judgment, and ensuring the ethical and responsible use of AI technology in the field of cardiac electrophysiology.
FREE course for EP Professionals.
Try another Blog by Steph Jasa!
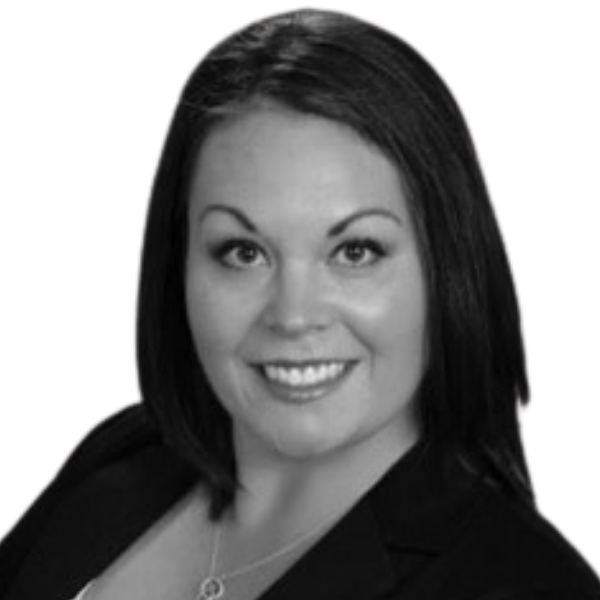
Steph Jasa
MA, MHA, CEPS, RCES, RCIS
Steph serves as the #chiefNERD for the CHART team. She has more than 20 years of experience in cardiology, including time in the hospital setting, industry, and consulting for cardiology companies. Her passion for providing education and training is underpinned by a desire to take complex concepts and make them easy to understand and apply in the clinical setting.
Connect with Steph on LinkedIn for information about CHART Healthcare Academy, Cardiac Electrophysiology and Cardiac Device Clinic training and education programs.
References:
Bohr, A., & Memarzadeh, K. (Eds.). (2020). Artificial Intelligence in Healthcare. Academic Press.
Consoli, S., Reforgiato Recupero, D., & Petkovic, M. (Eds.). (2019). Data Science for Healthcare: Methodologies and Applications. Springer.
Feeny, A.K., et al. (2020). Artificial Intelligence and Machine Learning in Arrhythmias and Cardiac Electrophysiology. Circ. https://doi.org/10.1161/CIRCEP.119.007952
Quer, Giorgio,et. al., (2021). Machine Learning and the Future of Cardiovascular Care. JACC State-of-the-Art Review.
Seuss, D. (1975). Oh, the Places You’ll Go! Random House.
Sau, Arunashis, et al. Artificial intelligence-enabled electrocardiogram to distinguish cavotricuspid isthmus dependence from other atrial tachycardia mechanisms. European Heart Journal-Digital Health, 2022, vol. 3, no 3, p. 405-414.
Yu, J., Wang, X., Chen, X., & Guo, J. (2021). Automatic premature ventricular contraction detection using deep metric learning and KNN. Biosensors, 11(3), 69.
Comments